Canadian researchers found that quantitative ultrasound radiomics in late stage breast cancer patients accurately predicts tumor response to chemotherapy using texture derivatives in images via machine learning analysis.
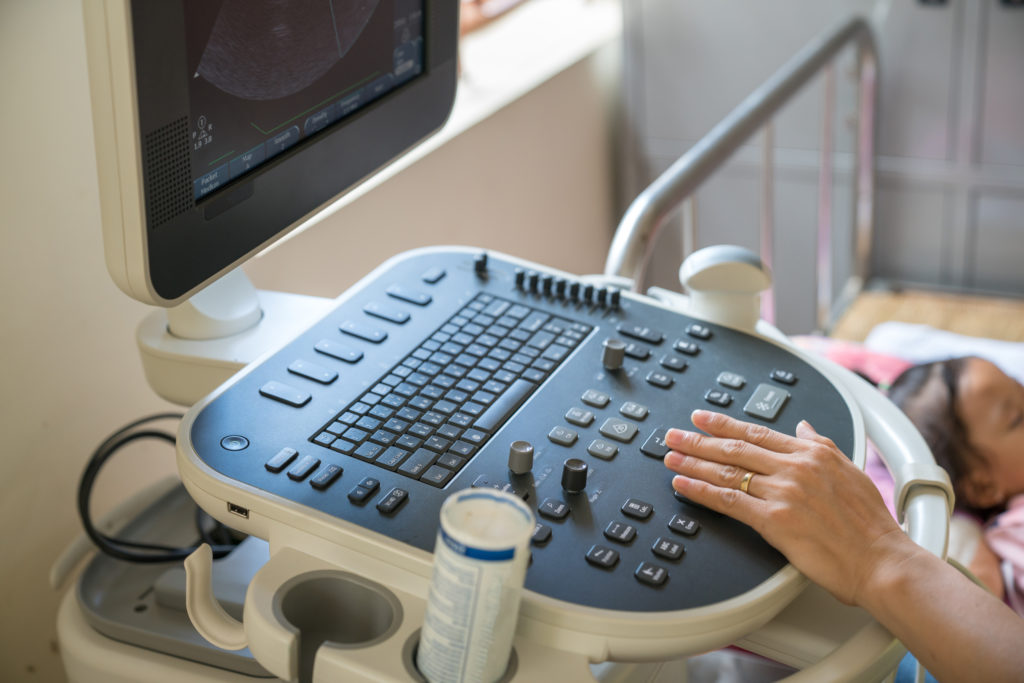
A team of multidisciplinary researchers from the University of Toronto, Sunnybrook Health Sciences Centre and Research Institute, and York University—all based out of Toronto, Canada—carried out a study that leads toward personalized oncology for later stage breast cancer patients.
The study found that computer-generated analysis of ultrasound images can detect tumor response to upcoming chemotherapy with high accuracy through texture derivative analysis using machine learning algorithms.
The Study
Imaging is an important step in current oncological practice. Various imaging instruments, such as MMG, USG, CT, MRI, and PET-CT, are used to manage patients with breast cancer in the diagnostic work up, staging, therapy guidance, response evaluation, and surveillance following treatment completion.
“Response monitoring during therapy provides a window for adopting personalized medicine.”
Since it has been shown that systemic therapies before surgery result in downsizing tumors and improving surgery outcomes, the team studied methods of improving quantitative ultrasound (QUS) before neo-adjuvant chemotherapy.
Researchers scanned 100 patients with locally advanced breast cancer (LABC) using QUS and computer generated analysis, radiomics, for texture derivative analysis to predict their responsiveness to chemotherapy treatment.
Five QUS parametric image-types were generated from radio-frequency data of the tumor mass. They used three machine learning algorithms (FLD, KNN, SVM) as their radiomic models to predict response and non-response to chemotherapy.
“As expected, clinical outcomes were distinctly different between the responders and non-responders.”
Conclusion
In summary, the researchers report that quantitative ultrasound radiomics can detect tumor response to neoadjuvant chemotherapy with high accuracy using texture derivative analysis of parametric images and a machine learning approach. The QUS-based model has the potential of identifying chemo-resistant tumors in patients ahead of time, improving treatment decisions.
“The KNN-model provided the best results with sensitivity, specificity, accuracy, and area under curve. The 5-year recurrence-free survival (RFS) calculated for KNN predicted responders and non-responders using QUS-Tex1-Tex2 model were comparable to RFS in the actual response groups.”
The team believes this is the first study to demonstrate the effectiveness of QUS texture derivatives applied to imaging data to predict the response (or lack of response) to preliminary chemotherapy in patients with advanced breast cancer. The researchers also say that early identification of patients not responding to neoadjuvant chemotherapy may have potentially significant clinical implications.
“Alternative chemotherapy regimens or upfront surgery should be considered in patients who are refractory to the standard protocols rather than continuing with the ineffective regimens.”
–
Oncotarget is a unique platform designed to house scientific studies in a journal format that is available for anyone to read – without a paywall making access more difficult. This means information that has the potential to benefit our societies from the inside out can be shared with friends, neighbors, colleagues and other researchers, far and wide.
Click here to read the full scientific paper, published in Oncotarget.